
4 Reasons to Add Mathematical Optimization to Your Analytics Toolbox




4 Reasons Why Data Scientists Should Add Mathematical Optimization to Their Analytics Toolbox
Â
Today’s data scientists need to have a full analytics toolbox at their disposal. But which tools do they actually need?
In addition to machine learning, visualization, heuristics, and other common tools, mathematical optimization is becoming an essential technology for more and more data scientists.
With a full set of analytics tools including mathematical optimization, data scientists can maximize the business value of their data–by using it make accurate predictions and optimal decisions
Indeed, mathematical optimization is a powerful prescriptive analytics technology that should be included in every data scientist’s analytics toolbox. Read this new management paper to find out why.
Customers
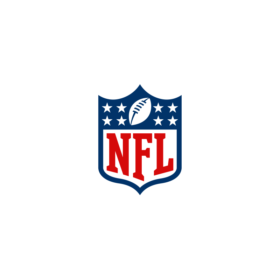
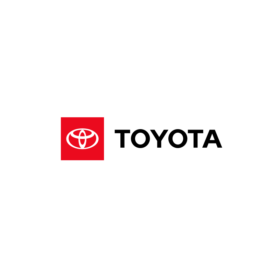
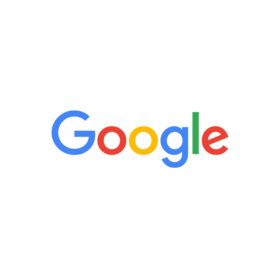
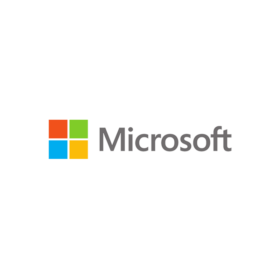
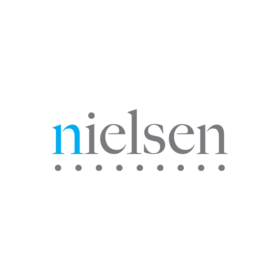
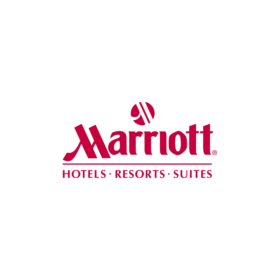
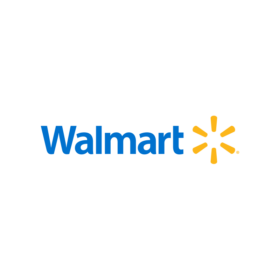
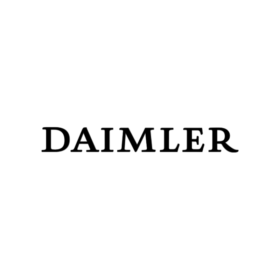
Why Should You Use Optimization?
The Next Step for Enterprises: Optimization Transformation
In this article, Gurobi Technical Fellow and VP Dr. Gregory Glockner details how organizations are applying mathematical optimization, a powerful prescriptive analytics technology, to power digital transformation, decision optimization, and competitive advantage.
Financial Services
Mathematical optimization is a well-established, essential technological tool in the financial services industry. For over 50 years, mathematical optimization technologies have been used by leading companies across the financial services ecosystem (including institutional and consumer banks, wealth management firms, hedge funds, insurance providers, and fintech players) to:
- Address a wide variety of complex business problems including portfolio optimization, cash management, trade settlement, and asset-liability management.
- Make optimal, data-driven decisions that deliver improved operational efficiency, profitability, and regulatory compliance as well as reduced risk and costs.
Â
Supply Chain
Leading companies across numerous industries use Gurobi’s mathematical optimization solver – in a wide variety of applications – to optimize their supply chain planning, decision making, and operations and keep supply and demand in balance.
With mathematical optimization, you can:
- Attain visibility and control over your end-to-end supply chain network.
- React and respond rapidly and effectively to changing conditions and disruptions across your supply chain.
- Make dynamic, data-driven decisions that optimize your company’s efficiency and profitability.
- Achieve your business goals by balancing cost and service-level tradeoffs – simultaneously satisfying customer demand and spurring bottom-line growth.
- Transform your supply chain from a source of costs into a source of competitive advantage.
Energy and Utilities
Gurobi solvers allow energy and utility companies to respond to the growing demand for services each year. Optimization enables organizations to delicately balance consumer utilization with responsible management of power generation and distribution. Optimization allows companies to turn data into insight by combining economic, social, and environmental considerations into a single mathematical model. Optimization can also be used to help companies mitigate risk and uncertainty in an increasingly competitive market.