Distributed Algorithms
As noted earlier, Gurobi Optimizer implements a number of distributed algorithms that allow you to use multiple machines to solve a problem faster. Available distributed algorithms are:
- A distributed MIP solver, which allows you to divide the work of solving a single MIP model among multiple machines. A manager machine passes problem data to a set of worker machines in order to coordinate the overall solution process.
- A distributed concurrent solver, which allows you to use multiple machines to solve an LP or MIP model. Unlike the distributed MIP solver, the concurrent solver doesn't divide the work among machines. Instead, each machine uses a different strategy to solve the whole problem, with the hope that one strategy will be particularly effective and will finish much earlier than the others. For some problems, this concurrent approach can be more effective than attempting to divide up the work.
- Distributed parameter tuning, which automatically searches for parameter settings that improve performance on your optimization model (or set of models). Tuning solves your model(s) with a variety of parameter settings, measuring the performance obtained by each set, and then uses the results to identify the settings that produce the best overall performance. The distributed version of tuning performs these trials on multiple machines, which makes the overall tuning process run much faster.
Distributed Workers and the Distributed Manager
Running distributed algorithms requires several machines. One acts as the manager, coordinating the activities of the set of machines. The others act as workers, receiving tasks from the manager. The manager typically acts as a worker itself, although not always. More machines generally produce better performance, although the marginal benefit of an additional machine typically falls off as you add more.
As we'll discuss shortly, distributed workers do not require Gurobi licenses. You can add any machine to a Remote Services cluster to act as a distributed worker. The manager does require a distributed algorithm license (you'll see a DISTRIBUTED= line in your license file if distributed algorithms are enabled).
A typical distributed optimization will look like the following, with all machines belonging to the same Remote Services cluster:
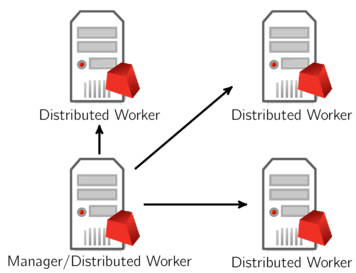
Another option is to use a machine outside of your Remote Services cluster as the manager:
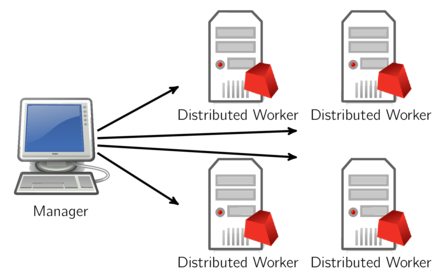
Note that we only allow a machine to act as manager for a single distributed job. If you want to run multiple distributed jobs simultaneously, you'll need multiple manager machines.
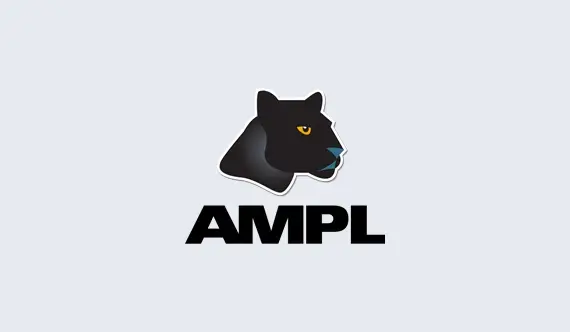

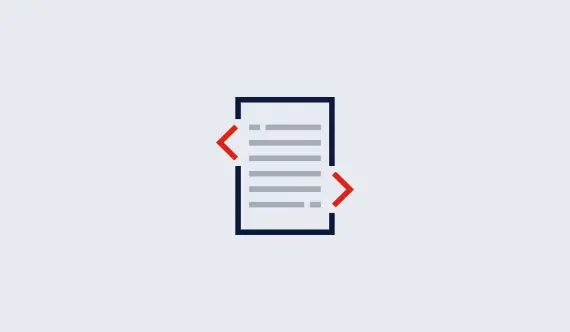
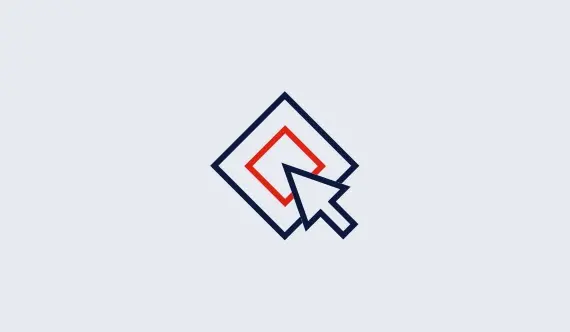
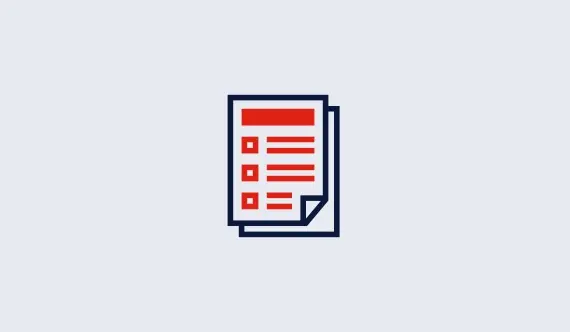
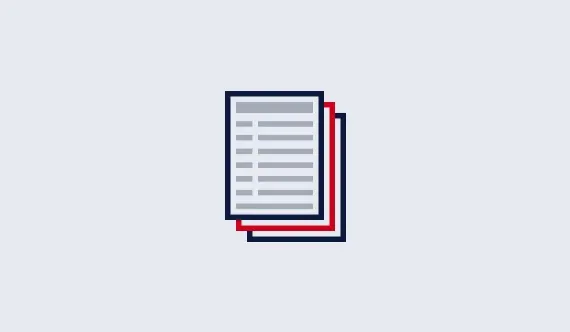
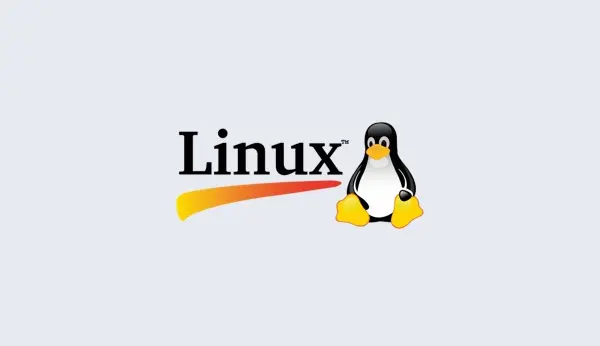
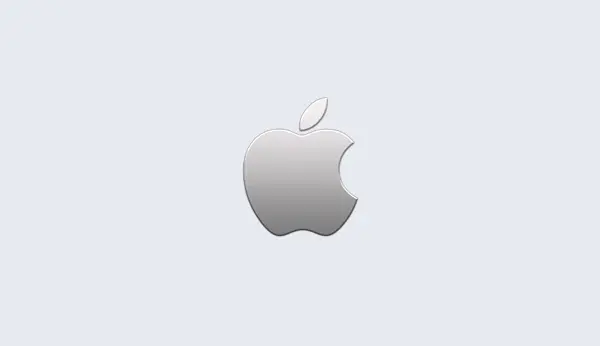
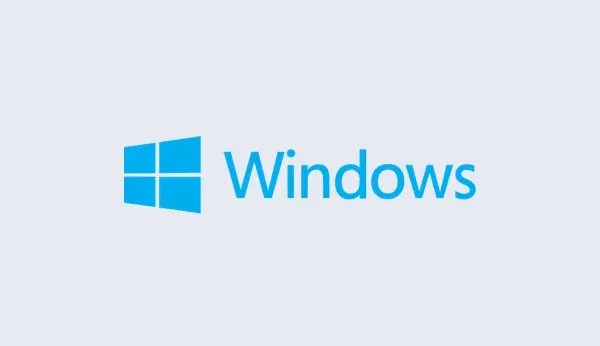